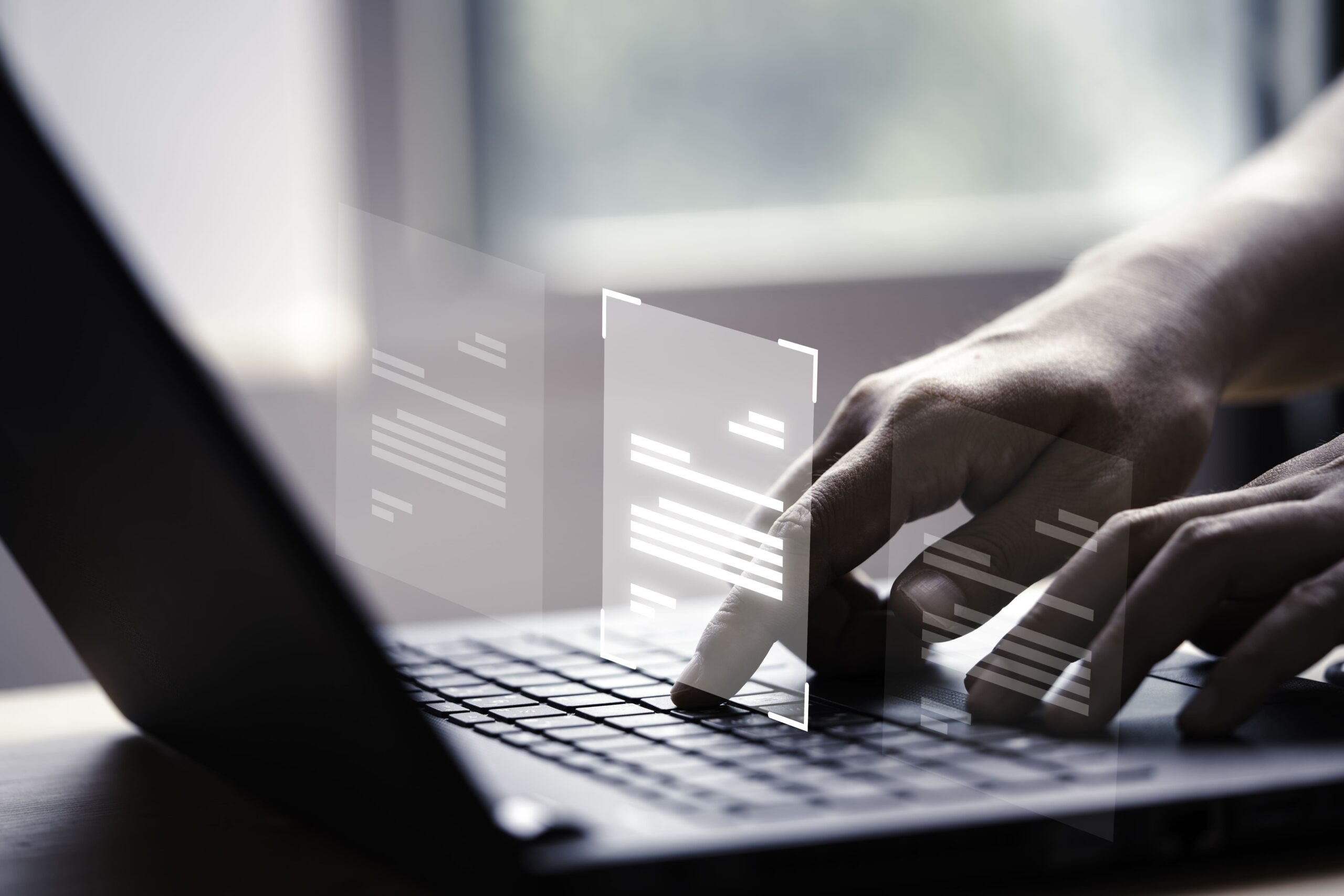
Key Points :
- Data cleaning ensures accuracy and reliability by identifying and rectifying errors, inconsistencies, and inaccuracies in datasets.
- Challenges with unclean data include duplicate entries, missing values, inaccuracies, outliers, irrelevant data, and collection errors.
- Effective data cleaning involves removing duplicates, handling missing values, validating data, standardizing formats, correcting errors, and documenting the process for transparency and reproducibility.
The business world is swimming in data these days. From giant factories to your corner store, everyone’s going digital. This means they’re carefully recording every sale and interaction in their computers. As a result, businesses end up with a giant pile of valuable information, just waiting to be analyzed and turned into useful insights. But here’s the catch: the quality of this information is super important. Clean data, which means it’s accurate, consistent, and complete, is like the magic key to unlocking powerful tools. It doesn’t just help with regular data analysis, it also helps build those super-smart AI models everyone’s talking about.
What is Data Cleaning ?
Data cleaning tackles the important task of identifying and fixing errors, inconsistencies, and inaccuracies in a dataset. This process involves a series of steps to make sure the data used for analysis or decisions isn’t messed up by anything that might throw off its accuracy.
The common problems faced with unclean data are as follows:
- Duplicate Data: Redundancy in datasets undermines analysis accuracy.
- Incomplete Data: Missing values disrupt analysis continuity and lead to inaccurate conclusions.
- Inaccurate Data: Errors, inconsistencies, and unreliable sources affect analysis precision.
- Outliers: Data points significantly deviating from the dataset’s pattern bias analysis results.
- Irrelevant Data: Inclusion of unnecessary data wastes resources and complicates analysis.
- Invalid Correlation: Misinterpreting variable relationships results in ineffective strategies.
- Collection Errors: Issues during data collection, like sampling errors or biases, affect data validity.
In the data cleaning process, begin by identify and address issues such as duplication, incompleteness, or inaccuracies. Remove duplicate entries to reduce redundancy, and handle missing values through imputation or deletion as necessary. Validate the data to ensure alignment with expectations and standardize formats for consistency. Correct errors, analyze outliers, and ensure categorical consistency. Normalize data for uniform comparison if needed, and filter out irrelevant data. Finally, double check the cleaned data and thoroughly document the cleaning process, including the steps taken and decisions made, to ensure transparency and reproducibility.
What are the benefits of clean data for a business?
Data is the heart of any successful business. But it’s clean data, specifically, that fuels informed decision-making. This reliable information allows businesses to develop accurate strategies and conduct insightful analysis. As a result, businesses can identify trends and optimize operations, ultimately driving efficiency and reducing costs.
Clean data goes beyond internal processes. It’s the key to unlocking customer preferences. By leveraging this knowledge, businesses can build stronger customer relationships by offering tailored products and services that meet evolving needs. Moreover, clean data ensures compliance with regulations and safeguards valuable customer information.
In short, clean data is a strategic asset. It propels businesses towards long-term success and growth.
The importance of clean data highlights its role as the bedrock of informed decision making and strategic planning. It instills confidence in leaders and is vital for staying competitive. Investing in data cleaning is essential for seizing opportunities, improve efficiency, and deliver exceptional customer service. In today’s digital era, clean data is crucial for organization success and relevance.